Big Data and Machine Learning for Materials
Date: Tuesday, March 13
Time: 8:30 a.m. to Noon
Location: Phoenix Convention Center, Room 129B
Sponsored by: TMS Materials Innovation Committee
Organizers: Carelyn Campbell, National Institute of Standards and Technology; Katsuyo Thornton, University of Michigan
Featured Speakers
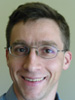
Jed W. Pitera, IBM Research – Almaden, USA
"Big Data for Materials R&D, Deployment, and Lifecycle"
In this talk, Pitera will give a broad overview of big data in the context of materials research & development. Different approaches to accelerate materials discovery all generate a wealth of data, including literature mining, computational experiments, multi-physics modeling and high-throughput experiments. By structuring and analyzing this data appropriately, we can support materials scientists with information systems that aid the search for new materials or new functions and that enhance collaboration. Our research group at IBM has a long-term exploratory project developing software to automatically extract and organize materials data. Some of the special challenges presented by materials information extraction will be highlighted. Further, the role of data in materials science is not limited to discovery. Data from materials in use offers additional opportunities and challenges that will also be discussed.
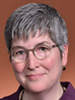
Elizabeth A. Holm, Carnegie Mellon University, USA
"How Materials Science Can Capitalize on Advances in Computer Science through Data Science and Machine Learning"
Computer science has advanced rapidly in the internet era, primarily due to the availability of very large data sets coupled with deep learning algorithms. The results have been impressive: autonomous vehicles on the streets of Pittsburgh, targeted advertisements on every web page, and computers besting humans at Jeopardy. However, although there are significant opportunities to apply data science to materials problems, materials science has not yet capitalized on these advances. In this talk, we will discuss case studies that involve using computer science approaches such as computer vision, data science, and machine learning to accomplish materials science and engineering objectives in interface science, micromechanics, microstructural characterization, and additive manufacturing. Based on our experience, we will suggest strategies for developing a data science ecosystem that combines advanced computational methods with large, well-curated data sets in order to realize the potential of these powerful tools.
Laura Biven, U.S. Department of Energy, USA
“Perspectives on Data Intensive Science from the DOE Office of Science”
The Department of Energy’s Office of Science is a basic research funding organization that delivers scientific discoveries and major scientific tools to transform our understanding of nature and advance the energy, economic, and national security of the United States. Integral to this mission is the management of large-scale scientific user facilities that are available for external use to advance scientific or technical knowledge. Examples of such facilities include light sources, particle colliders, super computers, fusion experiments, and environmental monitoring stations. Collectively, these facilities are expected to produce exabytes of data in the near future. This presentation will give an overview of the challenges and opportunities arising from this data deluge and how computing and, in several cases, machine learning will play an integral role.
Ian Foster, University of Chicago and Argonne National Laboratory, USA
"Going Smart and Deep on Materials"
As we acquire large quantities of science data from experiment and simulation, it becomes possible to apply machine learning (ML) to build predictive models and to guide future experiments. We thus need to make it easy to assemble, navigate, and compute on large data collections; develop, deploy, and run associated ML models; apply models to various tasks; and evaluate results. I describe here how we are realizing such capabilities within the NIST-supported Materials Data Facility (MDF) and the associated Deep Learning Hub (DLHub). I also report on several applications, including studies in which data from multiple labs are combined to produce ML models with enhanced accuracy, and experiments in which ML models are employed to manage supercomputer computations, improving accuracy and reducing cost.